Resumes
Resumes
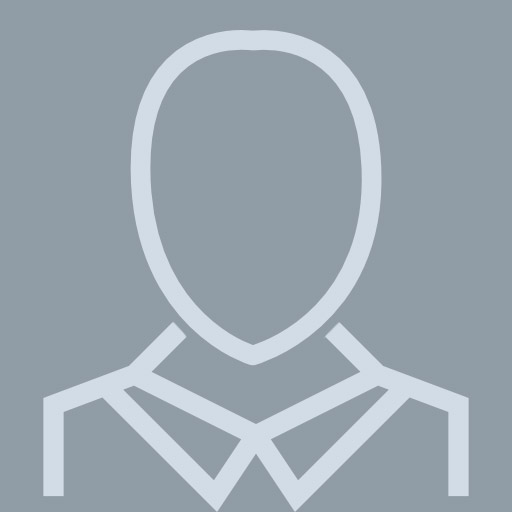
Owner, Automated Detection Systems, Inc
View pageLocation:
Los Angeles, CA
Industry:
Computer Software
Work:
Us Department of Homeland Security 2007 - 2008
Program Manager
Automated Detection Systems 2007 - 2008
Owner, Automated Detection Systems, Inc
Program Manager
Automated Detection Systems 2007 - 2008
Owner, Automated Detection Systems, Inc
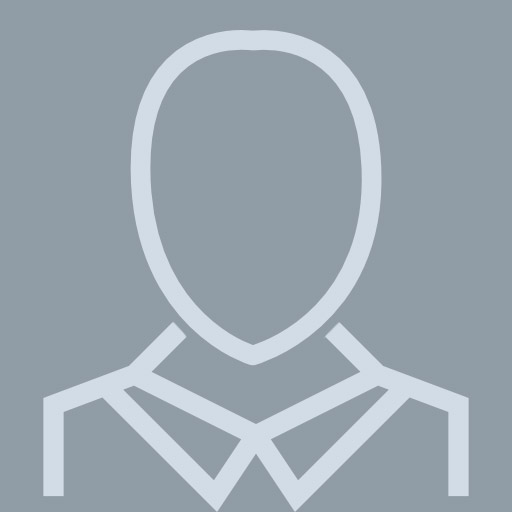
Narbik Manukian
View pageLocation:
Los Angeles, CA
Industry:
Research
Skills:
Developing Novel Mathematical and Algorithmic Solutions
Leading and Managing Research and Development Group
Creating New Business Opportunities and Obtaining New Contracts
Strong Communicative and Interaction Skills
Ts Clearance
Integration
Physics
Algorithms
Pattern Recognition
Software Engineering
Signal Processing
Radar
Image Processing
Testing
Security Clearance
Systems Engineering
Engineering
System Architecture
Leading and Managing Research and Development Group
Creating New Business Opportunities and Obtaining New Contracts
Strong Communicative and Interaction Skills
Ts Clearance
Integration
Physics
Algorithms
Pattern Recognition
Software Engineering
Signal Processing
Radar
Image Processing
Testing
Security Clearance
Systems Engineering
Engineering
System Architecture