Resumes
Resumes
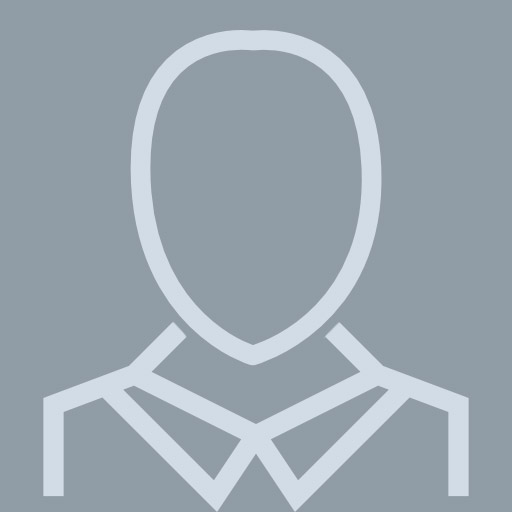
Deputy Managing Director Of Jd Ai Research, Technical Vice Preseident
View pageLocation:
Seattle, WA
Industry:
Computer Software
Work:
Jd.com
Deputy Managing Director of Jd Ai Research, Technical Vice Preseident
Microsoft 2017 - 2018
Principal Researcher and Research Manager
Microsoft 2006 - 2018
Principal Researcher and Affiliate Professor at U of Washington
University of Washington 2006 - 2018
Affiliate Professor
Microsoft Sep 2003 - May 2006
Software Development Engineer
Deputy Managing Director of Jd Ai Research, Technical Vice Preseident
Microsoft 2017 - 2018
Principal Researcher and Research Manager
Microsoft 2006 - 2018
Principal Researcher and Affiliate Professor at U of Washington
University of Washington 2006 - 2018
Affiliate Professor
Microsoft Sep 2003 - May 2006
Software Development Engineer
Education:
University of Missouri - Columbia 1999 - 2003
Doctorates, Doctor of Philosophy, Computer Science Chinese Academy of Sciences 1996 - 1999
Masters Tsinghua University 1991 - 1996
Bachelors Chinese Academy of Sciences 1982 - 1984
Masters
Doctorates, Doctor of Philosophy, Computer Science Chinese Academy of Sciences 1996 - 1999
Masters Tsinghua University 1991 - 1996
Bachelors Chinese Academy of Sciences 1982 - 1984
Masters
Skills:
Natural Language Processing
Machine Learning
Pattern Recognition
Artificial Intelligence
Speech Recognition
Information Retrieval
Data Mining
Text Mining
Information Extraction
Software Development
Computational Linguistics
Machine Translation
Java
Computer Vision
Signal Processing
Big Data
Mapreduce
Human Computer Interaction
Machine Learning
Pattern Recognition
Artificial Intelligence
Speech Recognition
Information Retrieval
Data Mining
Text Mining
Information Extraction
Software Development
Computational Linguistics
Machine Translation
Java
Computer Vision
Signal Processing
Big Data
Mapreduce
Human Computer Interaction
Languages:
English
Mandarin
Mandarin
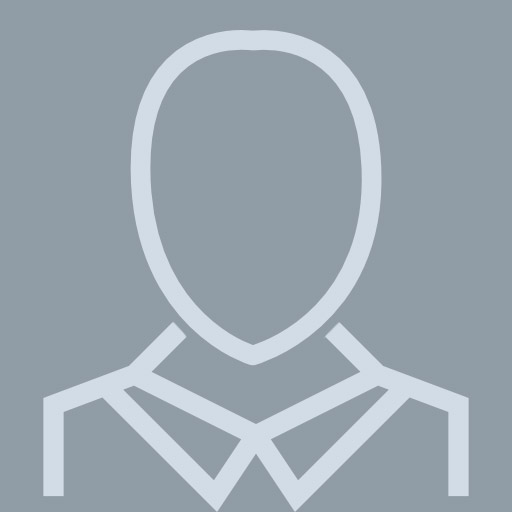
Researcher At Microsoft Research - Redmond
View pagePosition:
Researcher at Microsoft, Affiliate Professor at University of Washington
Location:
Greater Seattle Area
Industry:
Computer Software
Work:
Microsoft
Researcher
University of Washington - Greater Seattle Area since 2012
Affiliate Professor
Avaya Jun 2001 - Sep 2001
Intern
Researcher
University of Washington - Greater Seattle Area since 2012
Affiliate Professor
Avaya Jun 2001 - Sep 2001
Intern
Education:
University of Missouri-Columbia 1999 - 2003
PhD, Computer Science Chinese Academy of Sciences 1996 - 1999
Master, Singal and Information Processing Tsinghua University 1991 - 1996
Bachelor, Precision Instruments
PhD, Computer Science Chinese Academy of Sciences 1996 - 1999
Master, Singal and Information Processing Tsinghua University 1991 - 1996
Bachelor, Precision Instruments
Skills:
Natural Language Processing
Machine Learning
Speech Recognition
Information Retrieval
Pattern Recognition
Text Mining
Computational Linguistics
Information Extraction
Artificial Intelligence
Data Mining
Machine Translation
Machine Learning
Speech Recognition
Information Retrieval
Pattern Recognition
Text Mining
Computational Linguistics
Information Extraction
Artificial Intelligence
Data Mining
Machine Translation
Languages:
English
Chinese
Chinese